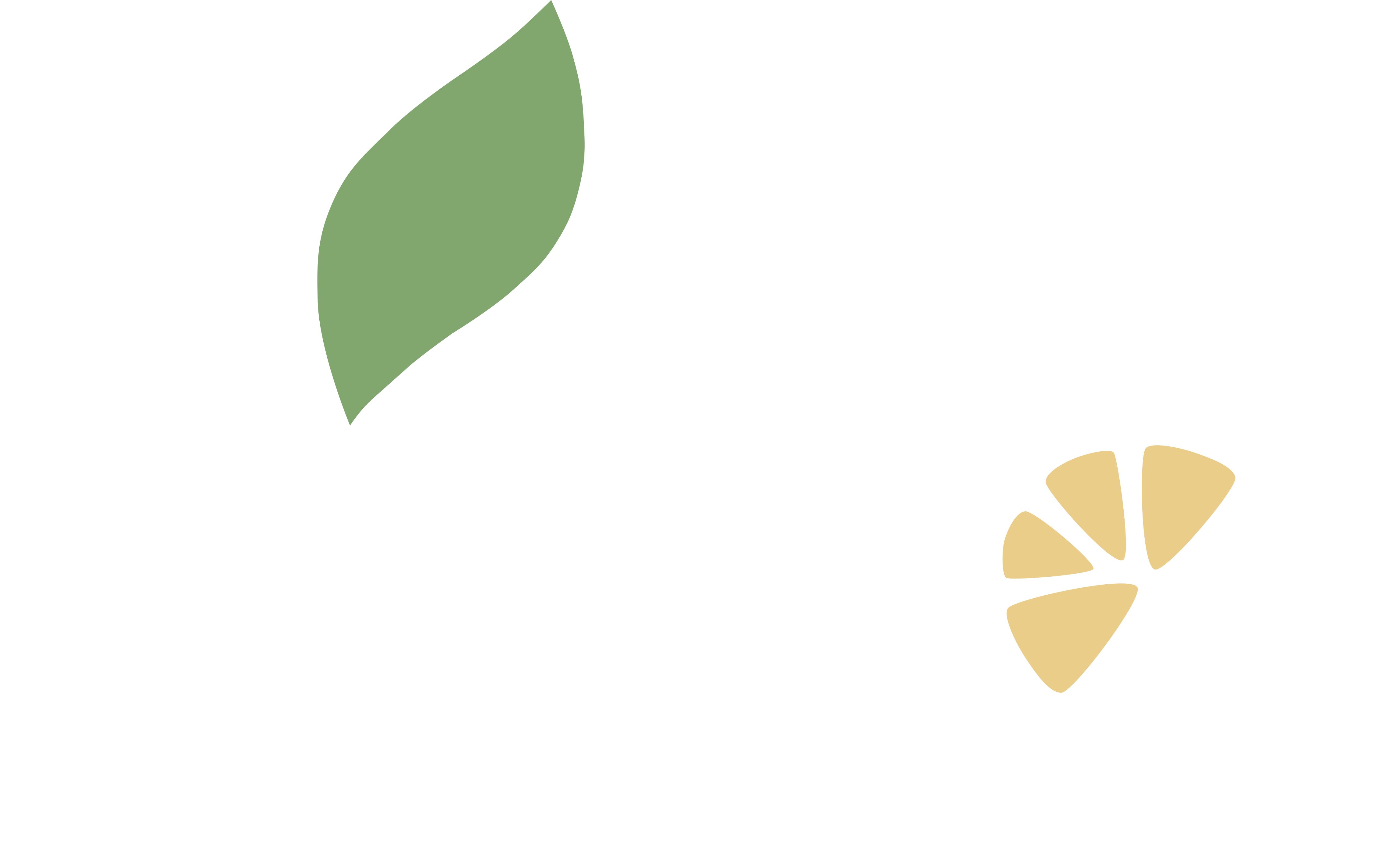
Ripe
I led our squad through a LEAN product process to find product-market fit with the least operational waste.
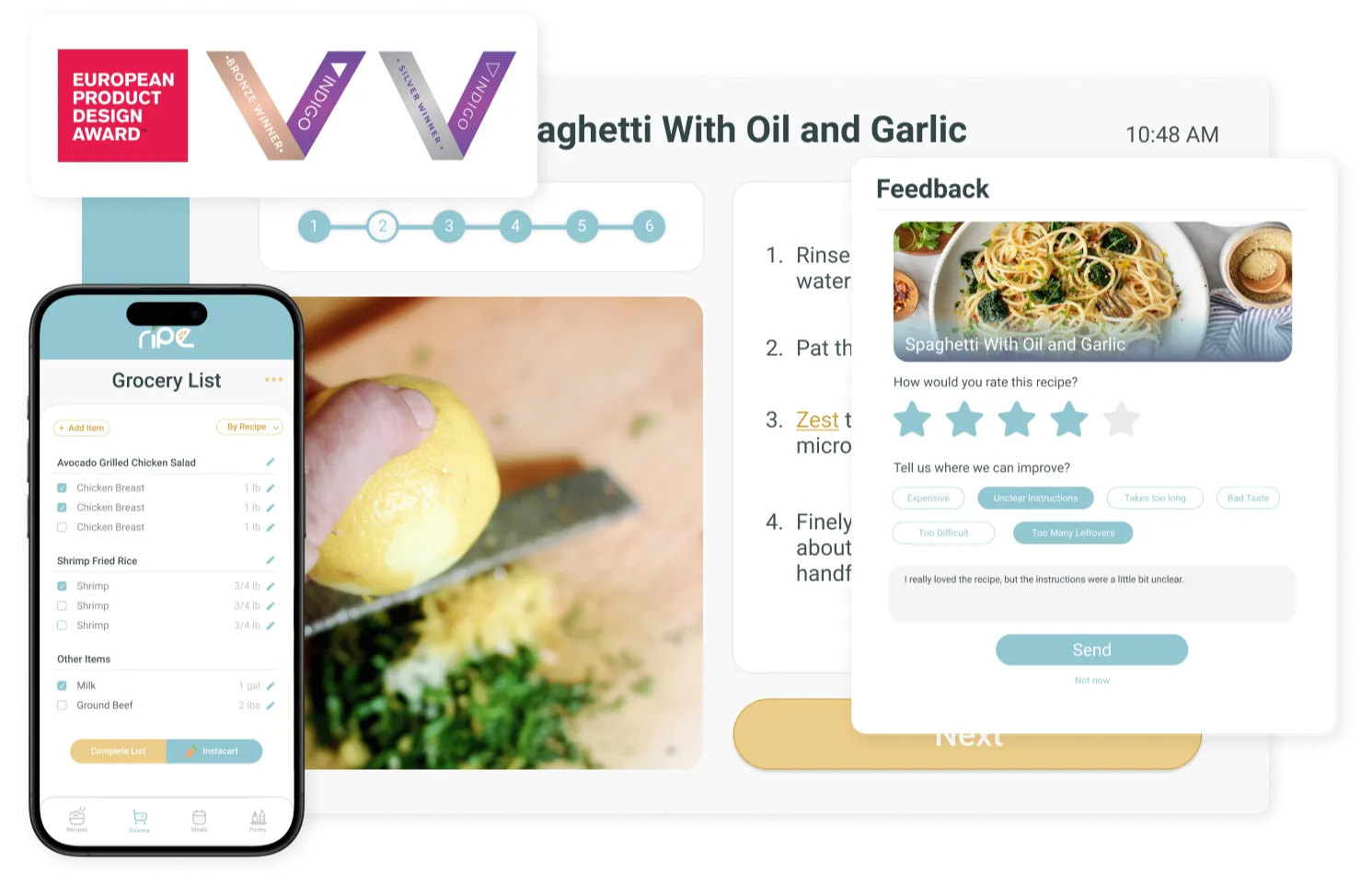
Project highlights
Designed and conducted product experiments that revealed increases in user-centric KPIs during product use.
Prototyped a machine learning algorithm to recommend recipes with a 74% case success rate.
Led lean discovery strategies to deliver value early and often.
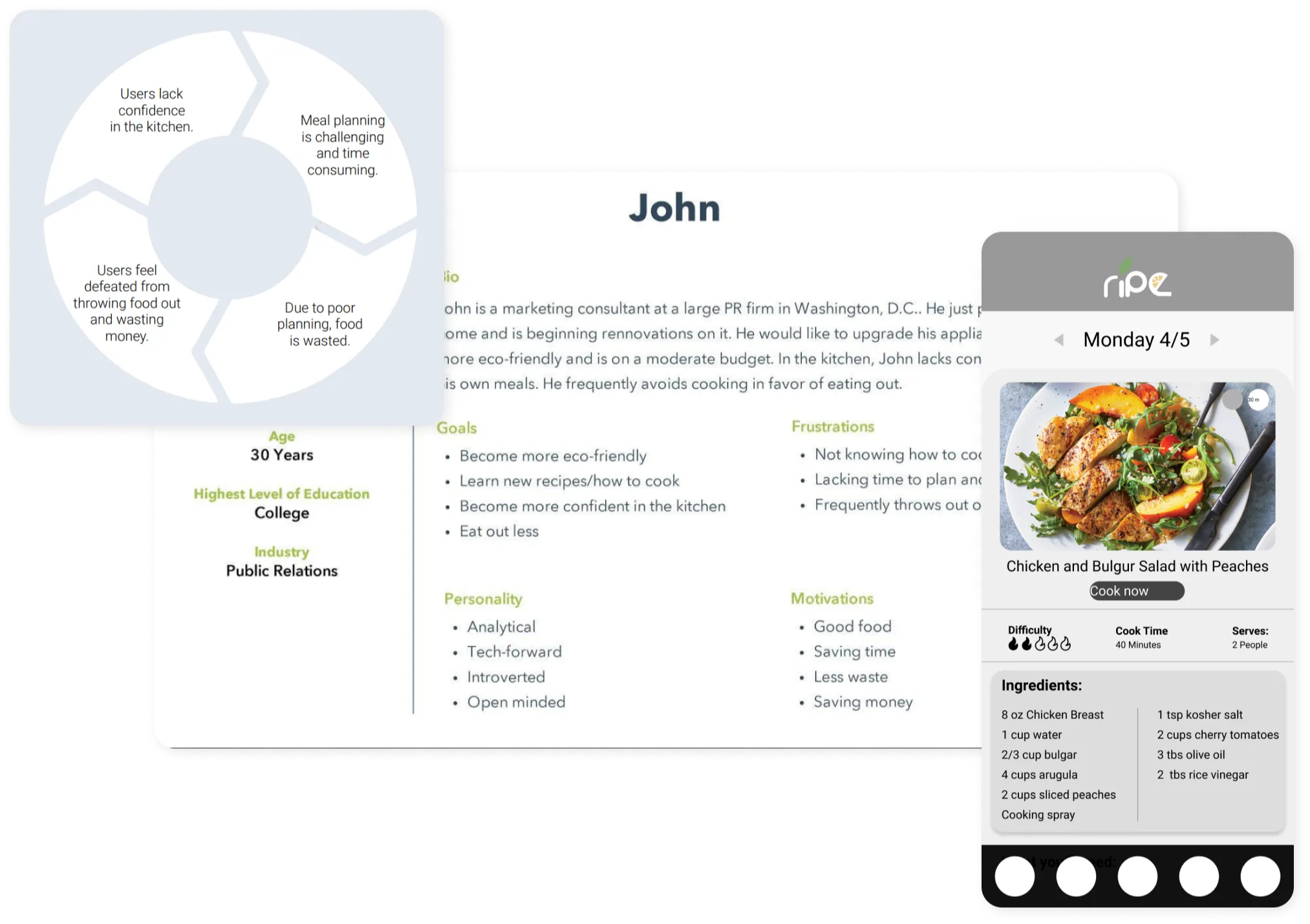
Discover the Product
Ripe is a mission-driven product: we each had a deep interest in making positive choices to help our communities and environment. Starting with research on our problem area, we identified food waste as a key, controllable concern, which we traced back to low confidence and poor planning in home kitchens. Our first prototypes started with this limited data then continued to grow as we continued to test and discover more context.
Tools Used
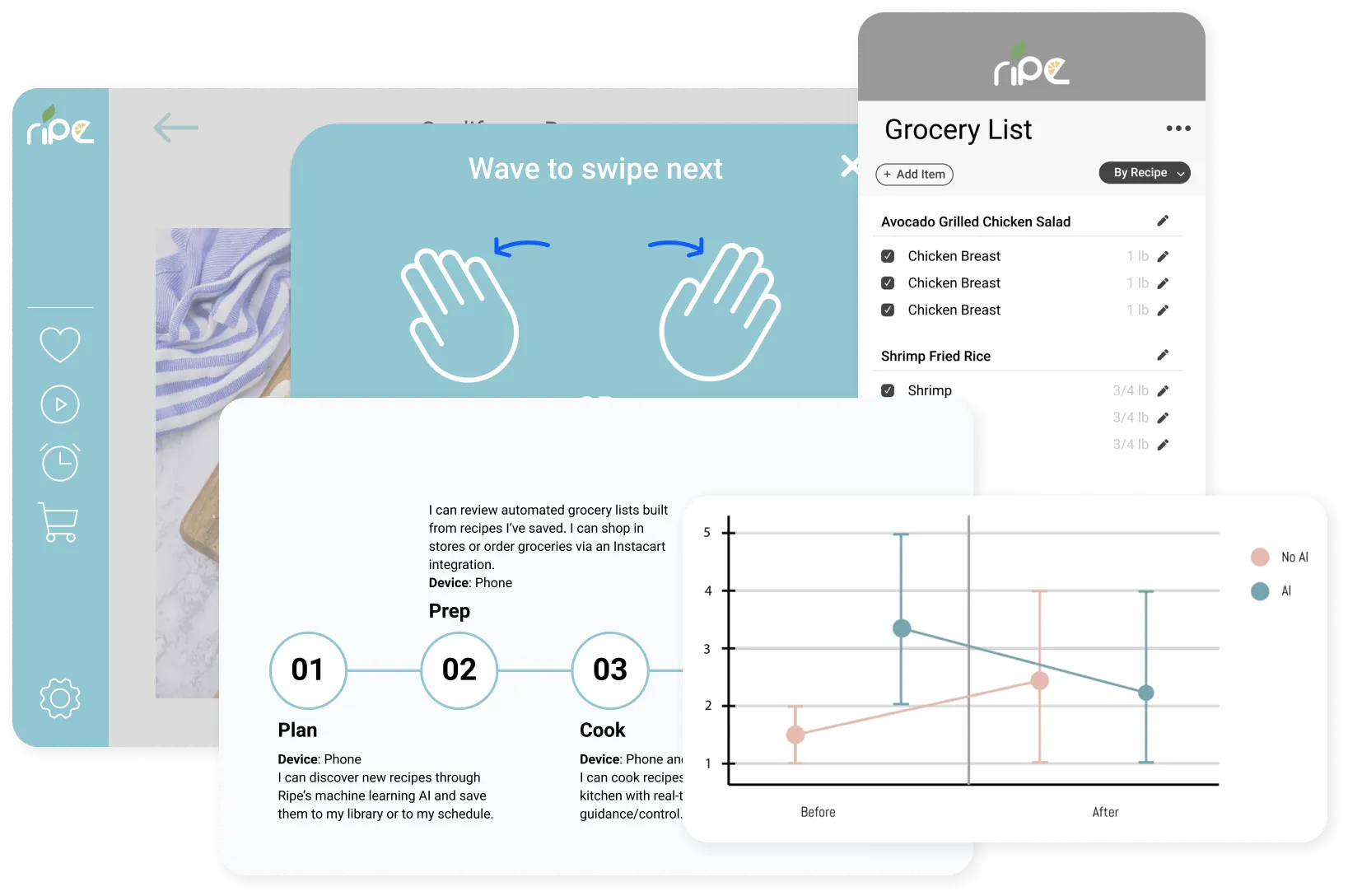
We built Ripe using a loose adaptation of scrum. Our squad created sprint plans, defined at a high level, assigned owners, then ran with it. This helped to break up the sometimes bulky feeling of building and researching in parallel, giving us more defined check-in points and goals to accomplish. We designed our tests with the same values as our product, focusing on achieving results with the least amount of work possible. That's how I ended up pretending to be a voice assistant AI, following the outputs of a prototyped machine-learning algorithm, talking a user tester through the process of selecting a recipe and cooking it on their own.
Tools Used
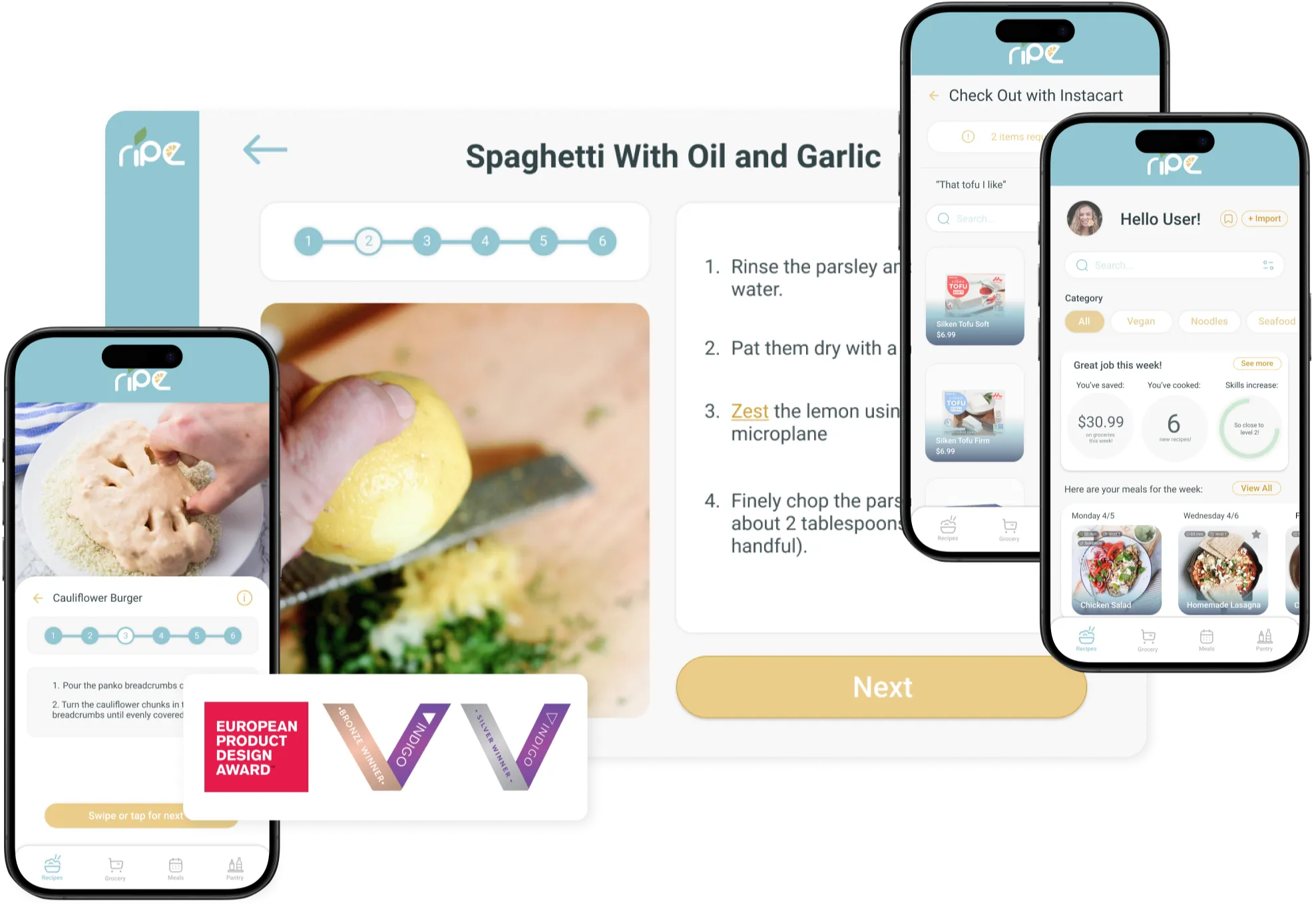
Deliver the Results
The wide array of testing techniques created constant data outputs that helped us to define our MVP completion. We found that after cooking with a Ripe prototype, our users were more confident, happier, and calmer than the control groups that cooked without. Test users also reported a higher understanding of the ingredients they cooked with. When combined with our algorithm's ability to suggest recipes based on user feedback, we successfully reduced our key problems: to help people find comfort and confidence in their home kitchens. We shared these results and received 20 Indigo Awards in graphic design, branding, product design, user experience, mobile design, and innovation, as well as becoming the Winner in Design for Society and Sustainability in the European Product Design Awards.
Tools Used
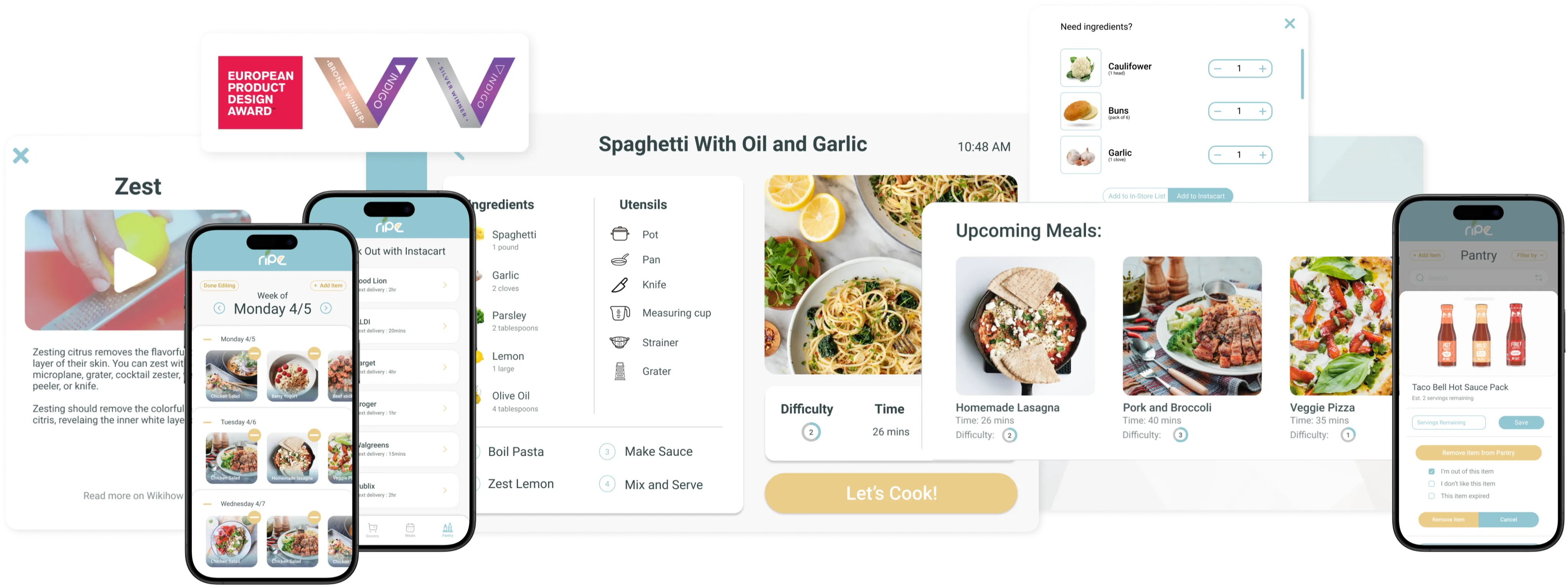